我有许多年鉴人像图片,正在尝试构建一个算法来检测这些人像。至少要正确检测矩形人像。示例1 示例2
我正在探索三个方向:
非常感谢任何关于矩形检测的帮助。 我开始使用Java和OpenCV 3编写代码。
以下是我应用于一张图片的代码:
在这一点上,我有如下结果:
尝试从上面的边缘中找到轮廓:
- 人脸检测
- 黑色矩形检测(因为肖像通常是在较亮的背景上的较暗形状中)
- 从OCR文本中提取人名
非常感谢任何关于矩形检测的帮助。 我开始使用Java和OpenCV 3编写代码。
以下是我应用于一张图片的代码:
System.loadLibrary(Core.NATIVE_LIBRARY_NAME);
Mat source = Imgcodecs.imread("Path/to/image", Imgcodecs.CV_LOAD_IMAGE_ANYCOLOR);
Mat destination = new Mat(source.rows(), source.cols(), source.type());
Imgproc.cvtColor(source, destination, Imgproc.COLOR_RGB2GRAY);
Imgproc.GaussianBlur(destination, destination, new Size(5, 5), 0, 0, Core.BORDER_DEFAULT);
int threshold = 100;
Imgproc.Canny(destination, destination, 50, 100);
Imgproc.Canny(destination, destination, threshold, threshold*3);
在这一点上,我有如下结果:
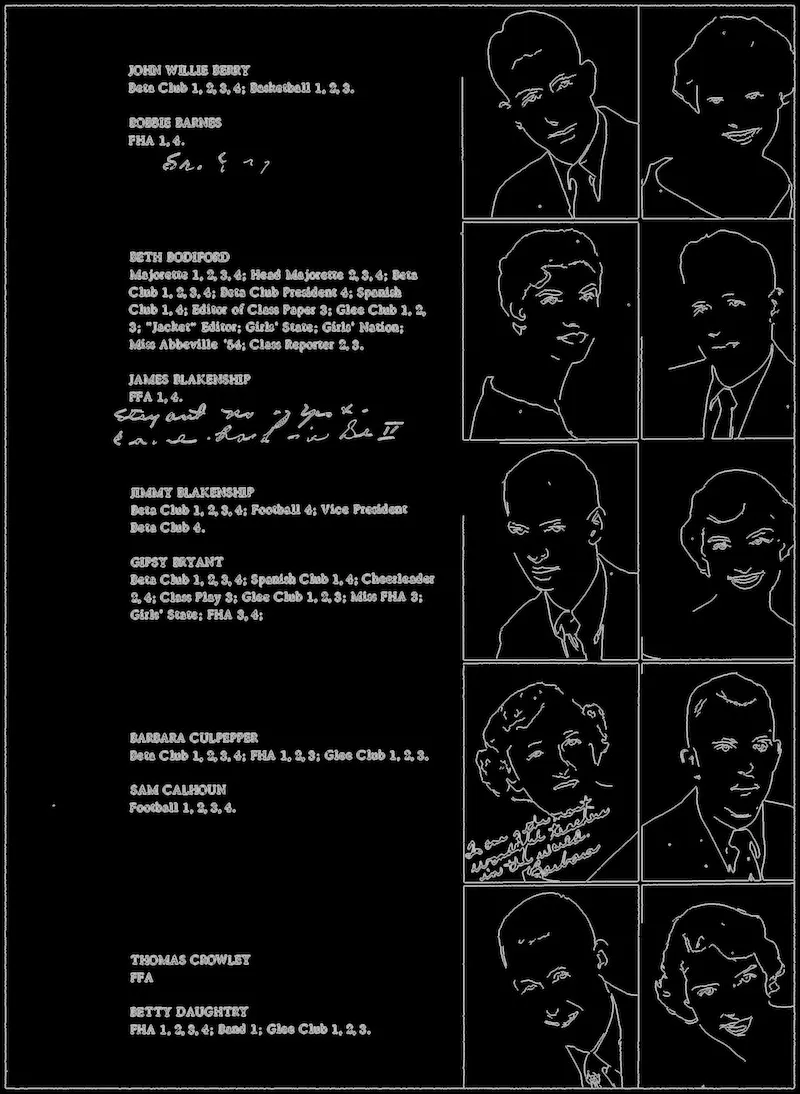
List<MatOfPoint> contourDetections = new ArrayList<>();
Mat hierarchy = new Mat();
// Find contours
Imgproc.findContours(destination, contourDetections, hierarchy, Imgproc.RETR_EXTERNAL, Imgproc.CHAIN_APPROX_SIMPLE);
// Draw contours
Imgproc.drawContours(source, contours, -1, new Scalar(255,0,0), 2);
但不确定如何从这些轮廓中提取矩形,因为许多线条是不完整的。
回到边缘并尝试使用HoughLinesP查找垂直和水平线:
Mat lines = new Mat();
int thre = 50;
int minLineSize = 250;
int lineGap = 80;
int ignoreLinesShorter = 300;
Imgproc.HoughLinesP(destination, lines, 1, Math.PI/180, thre, minLineSize, lineGap);
for(int c = 0; c < lines.rows(); c++) {
double[] vec = lines.get(c, 0);
double x1 = vec[0],
y1 = vec[1],
x2 = vec[2],
y2 = vec[3];
// Filtering only verticat and horizontal lines
if(x1 == x2 || y1 == y2) {
// Filtering out short lines
if(Math.abs(x1 - x2) > ignoreLinesShorter || Math.abs(y1 - y2) > ignoreLinesShorter) {
Point start = new Point(x1, y1);
Point end = new Point(x2, y2);
// Draw line
Imgproc.line(source, start, end, new Scalar(0,0,255), 2);
}
}
}
结果:
和等高线一样,我仍然没有看到正确的矩形可以检测。你能帮我指出正确的方向吗?也许有更简单的方法来执行这个任务吗?