我已经更深入地研究了这个问题,现在似乎最好的方法是直接从链接输出中创建一个新的函数来绘制径向聚类(而不是修改已有绘图)。我可能会尝试一些东西,但不会很快。
我假设您的数据自然适合这种径向嵌入。您是否已经验证过?linkage
中存在适合您目的的合适方法吗?
似乎对于任何方法,linkage
都会返回二叉树结构。在您的示例中,您拥有更通用的树。您需要一些额外的知识来合并树节点。这已经使修改原始树状图的想法失效了。
更新:
如果这个简单的示例绘图对您的目的来说足够相似,那么我将能够发布一些非常简单的代码来实现它。
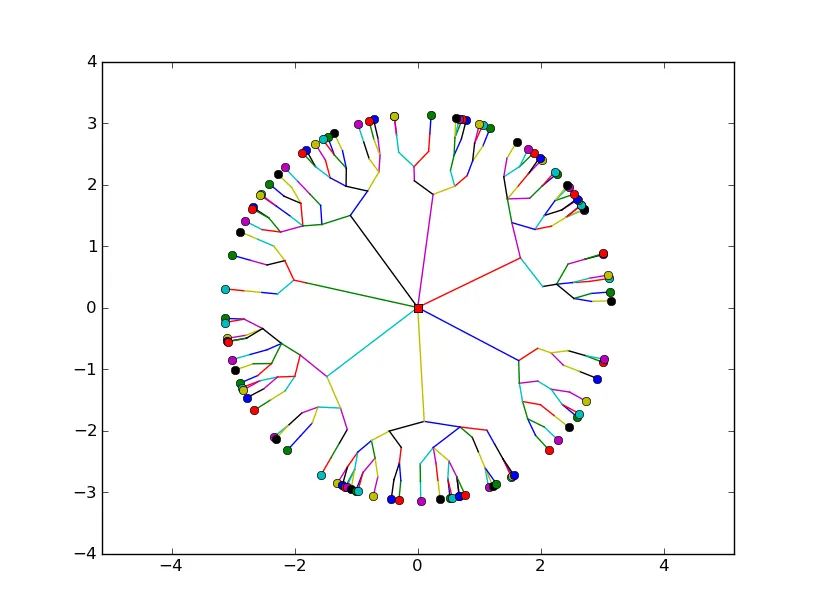
更新 2:
以下是代码:
radial_demo.py:
from numpy import r_, ones, pi, sort
from numpy.random import rand
from radial_grouper import tree, pre_order, post_order
from radial_visualizer import simple_link
from pylab import axis, figure, plot, subplot
def _s(sp, t, o):
subplot(sp)
t.traverse(simple_link, order= o)
axis('equal')
def demo1(n):
p= r_[2* pi* rand(1, n)- pi, ones((1, n))]
t= tree(p)
f= figure()
_s(221, t, pre_order)
_s(222, t, post_order)
t= tree(p, tols= sort(2e0* rand(9)))
_s(223, t, pre_order)
_s(224, t, post_order)
f.show()
if __name__ == '__main__':
demo1(123)
radial_grouper.py:
"""All grouping functionality is collected here."""
from collections import namedtuple
from numpy import r_, arange, argsort, array, ones, pi, where
from numpy import logical_and as land
from radial_support import from_polar
__all__= ['tree', 'pre_order', 'post_order']
Node= namedtuple('Node', 'ndx lnk')
def _groub_by(p, tol, r):
g, gm, gp= [], [], p- p[0]
while True:
if gp[-1]< 0: break
ndx= where(land(0.<= gp, gp< tol))[0]
if 0< len(ndx):
g.append(ndx)
gm.append(p[ndx].mean())
gp-= tol
return g, array([gm, [r]* len(gm)])
def _leafs(p):
return argsort(p[0])
def _create_leaf_nodes(ndx):
nodes= []
for k in xrange(len(ndx)):
nodes.append(Node(ndx[k], []))
return nodes
def _link_and_create_nodes(_n, n_, cn, groups):
nodes, n0= [], 0
for k in xrange(len(groups)):
nodes.append(Node(n_+ n0, [cn[m] for m in groups[k]]))
n0+= 1
return n_, n_+ n0, nodes
def _process_level(nodes, polar, p, tol, scale, _n, n_):
groups, p= _groub_by(p, tol, scale* polar[1, _n])
_n, n_, nodes= _link_and_create_nodes(_n, n_, nodes, groups)
polar[:, _n: n_]= p
return nodes, polar, _n, n_
def _create_tree(p, r0, scale, tols):
if None is tols:
tols= .3* pi/ 2** arange(5)[::-1]
_n, n_= 0, p.shape[1]
polar= ones((2, (len(tols)+ 2)* n_))
polar[0, :n_], polar[1, :n_]= p[0], r0
nodes= _create_leaf_nodes(_leafs(p))
nodes, polar, _n, n_= _process_level(
nodes, polar, polar[0, _leafs(p)], tols[0], scale, _n, n_)
for tol in tols[1:]:
nodes, polar, _n, n_= _process_level(
nodes, polar, polar[0, _n: n_], tol, scale, _n, n_)
polar[:, n_]= [0., 0.]
return Node(n_, nodes), polar[:, :n_+ 1]
def _simplify(self):
return self._root
def _call(self, node0, node1, f, level):
f(self, [node0.ndx, node1.ndx], level)
def pre_order(self, node0, f, level= 0):
for node1 in node0.lnk:
_call(self, node0, node1, f, level)
pre_order(self, node1, f, level+ 1)
def post_order(self, node0, f, level= 0):
for node1 in node0.lnk:
post_order(self, node1, f, level+ 1)
_call(self, node0, node1, f, level)
class tree(object):
def __init__(self, p, r0= pi, scale= .9, tols= None):
self._n= p.shape[1]
self._root, self._p= _create_tree(p, r0, scale, tols)
def traverse(self, f, order= pre_order, cs= 'Cartesian'):
self.points= self._p
if cs is 'Cartesian':
self.points= from_polar(self._p)
order(self, self._root, f, 0)
return self
def simplify(self):
self._root= _simplify(self)
return self
def is_root(self, ndx):
return ndx== self._p.shape[1]- 1
def is_leaf(self, ndx):
return ndx< self._n
if __name__ == '__main__':
from numpy import r_, round
from numpy.random import rand
from pylab import plot, show
def _l(t, n, l):
plot(t.points[0, n], t.points[1, n])
if 0== l:
plot(t.points[0, n[0]], t.points[1, n[0]], 's')
if t.is_leaf(n[1]):
plot(t.points[0, n[1]], t.points[1, n[1]], 'o')
n= 123
p= r_[2* pi* rand(1, n)- pi, ones((1, n))]
t= tree(p).simplify().traverse(_l)
show()
radial_support.py:
"""All supporting functionality is collected here."""
from numpy import r_, arctan2, cos, sin
from numpy import atleast_2d as a2d
def _a(a0, a1):
return r_[a2d(a0), a2d(a1)]
def from_polar(p):
"""(theta, radius) to (x, y)."""
return _a(cos(p[0])* p[1], sin(p[0])* p[1])
def to_polar(c):
"""(x, y) to (theta, radius)."""
return _a(arctan2(c[1], c[0]), (c** 2).sum(0)** .5)
def d_to_polar(D):
"""Distance matrix to (theta, radius)."""
pass
if __name__ == '__main__':
from numpy import allclose
from numpy.random import randn
c= randn(2, 5)
assert(allclose(c, from_polar(to_polar(c))))
radial_visualizer.py:
"""All visualization functionality is collected here."""
from pylab import plot
def simple_link(t, ndx, level):
"""Simple_link is just a minimal example to demonstrate what can be
achieved when it's called from _grouper.tree.traverse for each link.
- t, tree instance
- ndx, a pair of (from, to) indicies
- level, of from, i.e. root is in level 0
"""
plot(t.points[0, ndx], t.points[1, ndx])
if 0== level:
plot(t.points[0, ndx[0]], t.points[1, ndx[0]], 's')
if t.is_leaf(ndx[1]):
plot(t.points[0, ndx[1]], t.points[1, ndx[1]], 'o')
if __name__ == '__main__':
pass
您可以在
这里找到源代码。请随意修改它,但请确保将未来的修改与该要点同步。