在tcaswell的答案的基础上,您可以使用networkx的spring_layout
来排除标签,该算法实现了Fruchterman Reingold 力导向布局算法:
import matplotlib.pyplot as plt
import numpy as np
import networkx as nx
np.random.seed(2016)
xs = np.arange(10, step=0.1)+np.random.random(100)*3
ys = np.arange(10, step=0.1)+np.random.random(100)*3
labels = np.arange(100)
def repel_labels(ax, x, y, labels, k=0.01):
G = nx.DiGraph()
data_nodes = []
init_pos = {}
for xi, yi, label in zip(x, y, labels):
data_str = 'data_{0}'.format(label)
G.add_node(data_str)
G.add_node(label)
G.add_edge(label, data_str)
data_nodes.append(data_str)
init_pos[data_str] = (xi, yi)
init_pos[label] = (xi, yi)
pos = nx.spring_layout(G, pos=init_pos, fixed=data_nodes, k=k)
pos_after = np.vstack([pos[d] for d in data_nodes])
pos_before = np.vstack([init_pos[d] for d in data_nodes])
scale, shift_x = np.polyfit(pos_after[:,0], pos_before[:,0], 1)
scale, shift_y = np.polyfit(pos_after[:,1], pos_before[:,1], 1)
shift = np.array([shift_x, shift_y])
for key, val in pos.iteritems():
pos[key] = (val*scale) + shift
for label, data_str in G.edges():
ax.annotate(label,
xy=pos[data_str], xycoords='data',
xytext=pos[label], textcoords='data',
arrowprops=dict(arrowstyle="->",
shrinkA=0, shrinkB=0,
connectionstyle="arc3",
color='red'), )
all_pos = np.vstack(pos.values())
x_span, y_span = np.ptp(all_pos, axis=0)
mins = np.min(all_pos-x_span*0.15, 0)
maxs = np.max(all_pos+y_span*0.15, 0)
ax.set_xlim([mins[0], maxs[0]])
ax.set_ylim([mins[1], maxs[1]])
fig, ax = plt.subplots()
ax.plot(xs, ys, 'o')
repel_labels(ax, xs, ys, labels, k=0.0025)
plt.show()
产出。
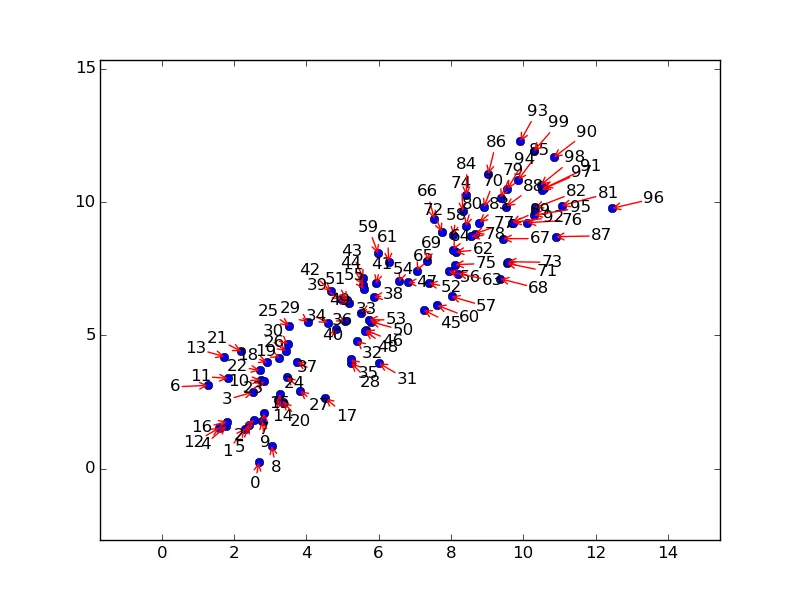