To plot a curve, you just need to define the relationship between response and predictor, and specify the range of the predictor value for which you'd like that curve plotted. e.g.:
dat <- structure(list(Response = c(1L, 1L, 1L, 1L, 1L, 1L, 1L, 1L, 1L,
1L, 1L, 1L, 1L, 1L, 1L, 1L, 1L, 1L, 1L, 1L, 0L, 0L, 0L, 0L, 0L,
0L, 0L), Temperature = c(29.33, 30.37, 29.52, 29.66, 29.57, 30.04,
30.58, 30.41, 29.61, 30.51, 30.91, 30.74, 29.91, 29.99, 29.99,
29.99, 29.99, 29.99, 29.99, 30.71, 29.56, 29.56, 29.56, 29.56,
29.56, 29.57, 29.51)), .Names = c("Response", "Temperature"),
class = "data.frame", row.names = c(NA, -27L))
temperature.glm <- glm(Response ~ Temperature, data=dat, family=binomial)
plot(dat$Temperature, dat$Response, xlab="Temperature",
ylab="Probability of Response")
curve(predict(temperature.glm, data.frame(Temperature=x), type="resp"),
add=TRUE, col="red")
curve(plogis(-88.4505 + 2.9677*x), min(dat$Temperature),
max(dat$Temperature), add=TRUE, lwd=2, lty=3)
legend('bottomright', c('temp.glm', 'Set 1'), lty=c(1, 3),
col=2:1, lwd=1:2, bty='n', cex=0.8)
In the second curve
call above, we are saying that the logistic function defines the relationship between x
and y
. The result of plogis(z)
is equivalent to that obtained when evaluating 1/(1+exp(-z))
. The min(dat$Temperature)
and max(dat$Temperature)
arguments define the range of x
for which y
should be evaluated. We don't need to tell the function that x
refers to temperature; this is implicit when we specify that the response should be evaluated for that range of predictor values.
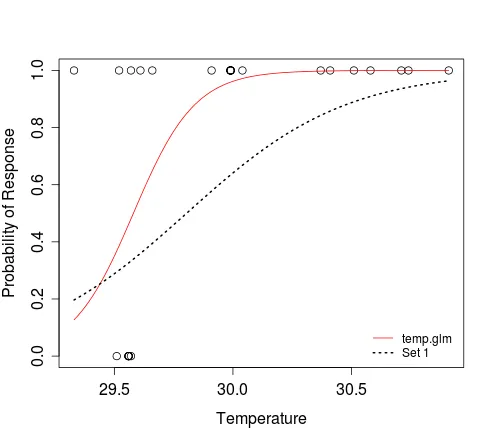
As you can see, the curve
function allows you to plot a curve without needing to simulate predictor (e.g. temperature) data. If you still need to do this, e.g. to plot some simulated outcomes of Bernoulli trials that conform to a particular model, then you can try the following:
n <- 100
temp <- runif(n, 27, 33)
sim.response <- function(alpha, beta) {
y <- sapply(temp, function(x) rbinom(1, 1, plogis(alpha + beta*x)))
plot(y ~ temp, pch=20, xlab='Temperature', ylab='Response')
curve(plogis(alpha + beta*x), min(temp), max(temp), add=TRUE, lwd=2)
return(y)
}
Examples:
y <- sim.response(-88.4505, 2.9677)
y <- sim.response(-88.585533, 2.972168)
y <- sim.response(coef(temperature.glm)[1], coef(temperature.glm)[2])
The figure below shows the plot produced by the first example above, i.e. results of a single Bernoulli trial for each value of the random vector of temperature, and the curve that describes the model from which the data were simulated.
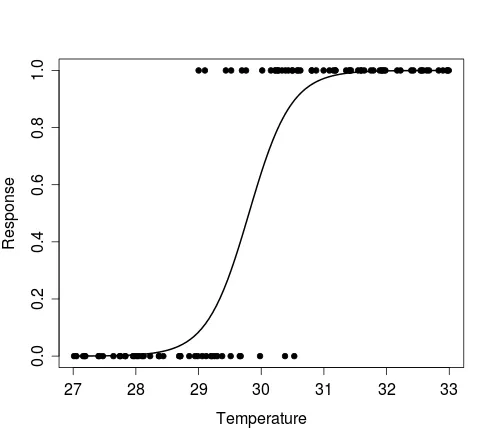
plot
调用之前使用par(mfrow=c(1, 2))
。否则,Richie的建议是调用两次curve
来叠加两条曲线。 - jbaumsrbinom(1000, 1, (1/(1+exp(-(-88.4505 + 2.9677*x))))
。然而,更简单的方法是rbinom(1000, 1, plogis(-88.4505 + 2.9677*x))
,请查看下面我的答案以获取更详细的解释。 - jbaums