你好,我试图使用Resnet神经网络对癌症数据集进行训练,采用微调方法。
以下是我的微调方法:
image_input = Input(shape=(224, 224, 3))
model = ResNet50(input_tensor=image_input, include_top=True,weights='imagenet')
model.summary()
last_layer = model.get_layer('avg_pool').output
x= Flatten(name='flatten')(last_layer)
out = Dense(num_classes, activation='softmax', name='output_layer')(x)
custom_resnet_model = Model(inputs=image_input,outputs= out)
custom_resnet_model.summary()
for layer in custom_resnet_model.layers[:-1]:
layer.trainable = False
custom_resnet_model.layers[-1].trainable
custom_resnet_model.compile(Adam(lr=0.001),loss='categorical_crossentropy',metrics=['accuracy'])
custom_resnet_model.summary()
tensorboard = TensorBoard(log_dir='./logs', histogram_freq=0,
write_graph=True, write_images=False)
hist = custom_resnet_model.fit(X_train, X_valid, batch_size=32, epochs=nb_epoch, verbose=1, validation_data=(Y_train, Y_valid),callbacks=[tensorboard])
(loss, accuracy) = custom_resnet_model.evaluate(Y_train,Y_valid,batch_size=batch_size,verbose=1)
print("[INFO] loss={:.4f}, accuracy: {:.4f}%".format(loss,accuracy * 100))
df = pd.read_csv('C:/CT_SCAN_IMAGE_SET/resnet_50/dbs2017/data/stage1_sample_submission.csv')
df2 = pd.read_csv('C:/CT_SCAN_IMAGE_SET/resnet_50/dbs2017/data/stage1_solution.csv')
x = np.array([np.mean(np.load('E:/224x224/%s.npy' % str(id)), axis=0) for id in df['id'].tolist()])
x = x.transpose(0,2,3,1)
# Make predictions
pred = model.predict(x, batch_size=batch_size, verbose=1) #predict(self, x, batch_size=None, verbose=0, steps=None)
print (pred)
我使用验证集来预测最终结果。 但即使进行了100个epoch,我的预测类别的概率仍然非常低。
5.41865666e-05 2.16298591e-04 2.77880055e-04 7.53038039e-05
6.03657216e-04 1.30494649e-04 4.92068466e-05 5.37877844e-04
1.61486780e-04 6.16881996e-04 9.92802554e-04 5.50923753e-04
3.62671199e-05 3.44127137e-03 7.17231014e-05 2.79643398e-04
2.86785862e-03 1.70384112e-04 6.59705256e-05 7.11611006e-04
2.09898906e-04 1.82953620e-04 8.88684444e-05 1.87824480e-04
1.32007655e-04 2.11239138e-04 7.63713342e-06 1.29785520e-04
1.09007429e-04 3.14327976e-04 4.73849563e-04 4.22359008e-04
6.27386966e-04 2.03593503e-04 1.72056989e-05 8.38911365e-05
1.91937244e-04 1.59160278e-04 5.24159847e-03 1.45429352e-04
4.30631888e-04 6.92744215e-04 1.00537611e-04 6.27409827e-05
3.87431937e-04 1.37840703e-04 1.04467930e-04 1.74013167e-05
1.18957250e-04 2.77637475e-04 2.25973461e-04 1.21678226e-04
2.42197304e-04 2.99750012e-04 1.16530759e-03 1.29382452e-03
7.35349662e-04 5.71311277e-04 1.26631945e-04 4.74024746e-05
3.71460657e-04 1.23646241e-04]
这是如何显示TensorBoard结果的。
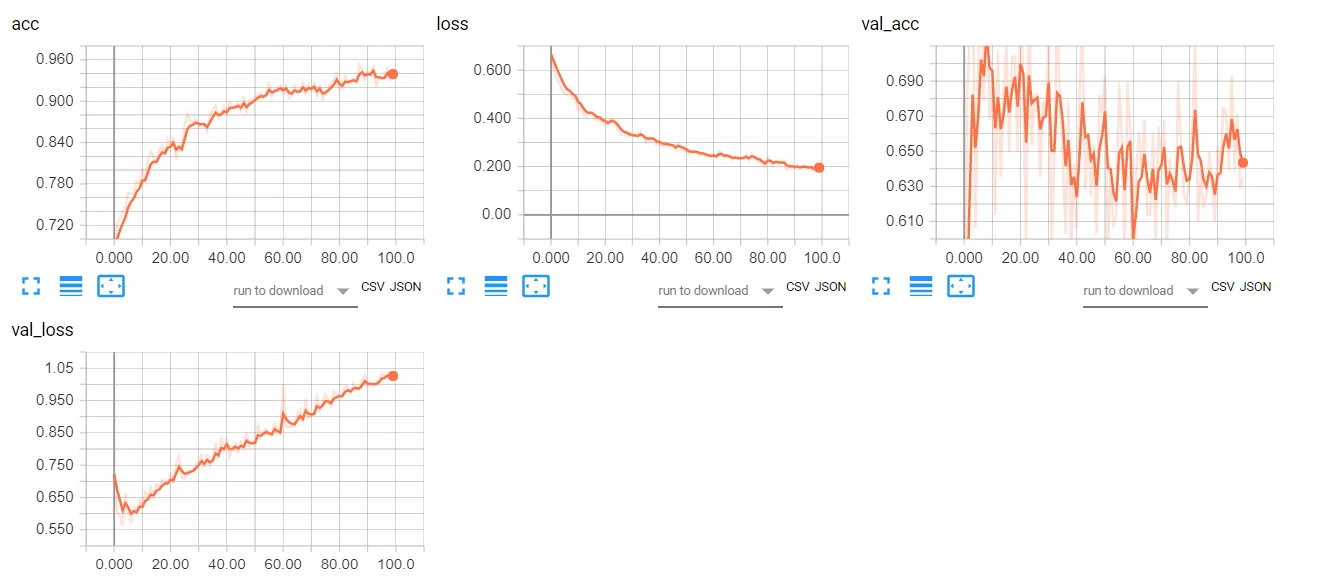
附:根据一个答案所建议的ResNet网络部分的摘要。
add_15 (Add) (None, 7, 7, 2048) 0 bn5b_branch2c[0][0]
activation_43[0][0]
__________________________________________________________________________________________________
activation_46 (Activation) (None, 7, 7, 2048) 0 add_15[0][0]
__________________________________________________________________________________________________
res5c_branch2a (Conv2D) (None, 7, 7, 512) 1049088 activation_46[0][0]
__________________________________________________________________________________________________
bn5c_branch2a (BatchNormalizati (None, 7, 7, 512) 2048 res5c_branch2a[0][0]
__________________________________________________________________________________________________
activation_47 (Activation) (None, 7, 7, 512) 0 bn5c_branch2a[0][0]
__________________________________________________________________________________________________
res5c_branch2b (Conv2D) (None, 7, 7, 512) 2359808 activation_47[0][0]
__________________________________________________________________________________________________
bn5c_branch2b (BatchNormalizati (None, 7, 7, 512) 2048 res5c_branch2b[0][0]
__________________________________________________________________________________________________
activation_48 (Activation) (None, 7, 7, 512) 0 bn5c_branch2b[0][0]
__________________________________________________________________________________________________
res5c_branch2c (Conv2D) (None, 7, 7, 2048) 1050624 activation_48[0][0]
__________________________________________________________________________________________________
bn5c_branch2c (BatchNormalizati (None, 7, 7, 2048) 8192 res5c_branch2c[0][0]
__________________________________________________________________________________________________
add_16 (Add) (None, 7, 7, 2048) 0 bn5c_branch2c[0][0]
activation_46[0][0]
__________________________________________________________________________________________________
activation_49 (Activation) (None, 7, 7, 2048) 0 add_16[0][0]
__________________________________________________________________________________________________
avg_pool (AveragePooling2D) (None, 1, 1, 2048) 0 activation_49[0][0]
__________________________________________________________________________________________________
flatten_1 (Flatten) (None, 2048) 0 avg_pool[0][0]
__________________________________________________________________________________________________
fc1000 (Dense) (None, 1000) 2049000 flatten_1[0][0]
__________________________________________________________________________________________________
dense_1 (Dense) (None, 512) 512512 fc1000[0][0]
__________________________________________________________________________________________________
dropout_1 (Dropout) (None, 512) 0 dense_1[0][0]
__________________________________________________________________________________________________
dense_2 (Dense) (None, 512) 262656 dropout_1[0][0]
__________________________________________________________________________________________________
dropout_2 (Dropout) (None, 512) 0 dense_2[0][0]
__________________________________________________________________________________________________
output_layer (Dense) (None, 2) 1026 dropout_2[0][0]
==================================================================================================